Multimodal Deep Learning
2023-01-11
Preface
Author: Matthias Aßenmacher
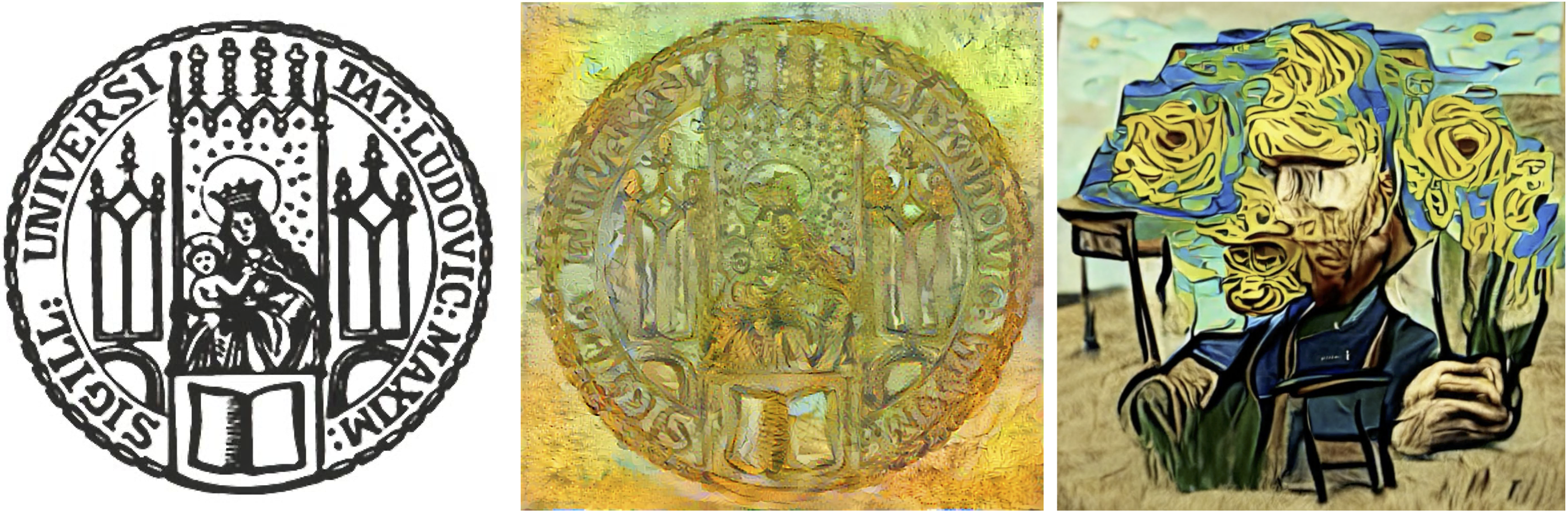
FIGURE 0.1: LMU seal (left) style-transferred to Van Gogh’s Sunflower painting (center) and blended with the prompt - Van Gogh, sunflowers - via CLIP+VGAN (right).
In the last few years, there have been several breakthroughs in the methodologies used in Natural Language Processing (NLP) as well as Computer Vision (CV). Beyond these improvements on single-modality models, large-scale multi-modal approaches have become a very active area of research.
In this seminar, we reviewed these approaches and attempted to create a solid overview of the field, starting with the current state-of-the-art approaches in the two subfields of Deep Learning individually. Further, modeling frameworks are discussed where one modality is transformed into the other Chapter 3.1 and Chapter 3.2), as well as models in which one modality is utilized to enhance representation learning for the other (Chapter 3.3 and Chapter 3.4). To conclude the second part, architectures with a focus on handling both modalities simultaneously are introduced (Chapter 3.5). Finally, we also cover other modalities (Chapter 4.1 and Chapter 4.2) as well as general-purpose multi-modal models (Chapter 4.3), which are able to handle different tasks on different modalities within one unified architecture. One interesting application (Generative Art, Chapter 4.4) eventually caps off this booklet.
Citation
If you refer to the book, please use the following citation (authors in alphabetical order):
@misc{seminar_22_multimodal,
title = {Multimodal Deep Learning},
author = {Akkus, Cem and Chu, Luyang and Djakovic, Vladana and
Jauch-Walser, Steffen and Koch, Philipp and Loss,
Giacomo and Marquardt, Christopher and Moldovan,
Marco and Sauter, Nadja and Schneider, Maximilian
and Schulte, Rickmer and Urbanczyk, Karol and
Goschenhofer, Jann and Heumann, Christian and
Hvingelby, Rasmus and Schalk, Daniel and
Aßenmacher, Matthias},
url = {https://slds-lmu.github.io/seminar_multimodal_dl/},
day = {30},
month = {Sep},
year = {2022}
}

Creative Commons License
This book is licensed under the Creative Commons Attribution-NonCommercial-ShareAlike 4.0 International License.